Fixing Gen AI trust issues: three focus areas to keep on your radar
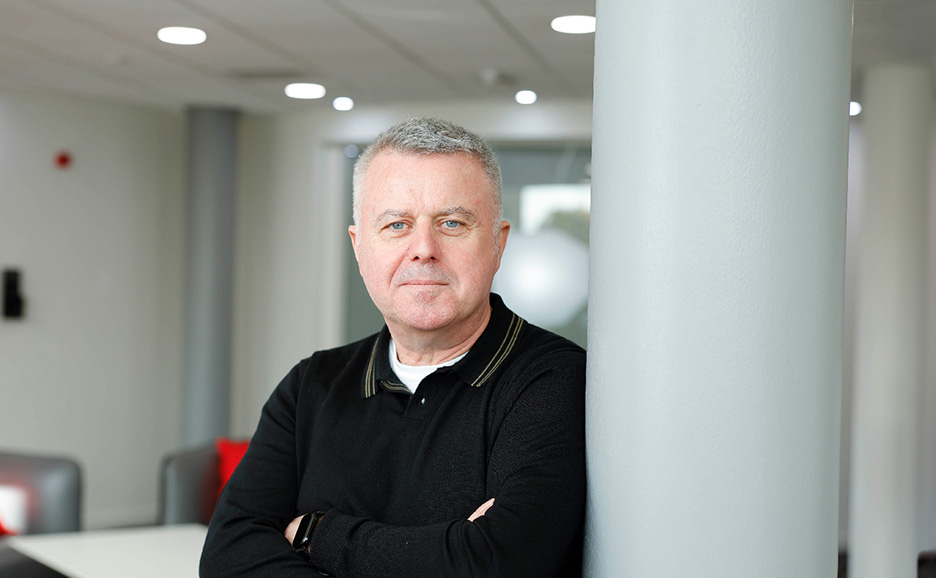
Article|2024-01-12
6 minute read
Leaders must focus on tackling generative AI’s trust and transparency issues before they can reap the business benefits of this game-changing technology, says John Walsh, CTO Europe at Fujitsu.
There’s no escaping the buzz around generative AI (Gen AI). The technology has massive transformative potential to benefit humankind – from making breakthroughs in, for example, environment and sustainability (predictive capability) to material science (creative capability) and personalized medicine (personalization capability).
Gen AI will be a powerful tool in augmenting business leaders ability to make smarter decisions because they can go beyond the usual practice of basing decisions on conventional datasets, regular reporting and historical trends. By infusing Gen AI models into decision-making processes, businesses can conduct deeper fact-finding exercises and experimental data-based simulations that can inspire new ideas and potentially ground-breaking possibilities.
But to avoid costly mistakes, decision-makers should be cognizant to the current limitations of Gen AI including: the accuracy of its output, security, with particular emphasis on personal or key company data, tracking of how results are derived (“explainability”) and growing calls for regulation (and eventual penalties for non-conformance).
To help your brand leverage this technology responsibly, here are three key trust and transparency principles to follow to ensure that Gen AI helps – not harms — your business, and wider society.
“Generative AI is a massively useful tool, but we need to understand its current constraints. My general advice around Gen AI is: do not blindly accept everything at face value.”
1. Beware blind trust in the technology
People might think Gen AI is a simple case of, “I type in a question, I get the answer.” But underneath the hood of this technology is a large and complex model that’s inherently opaque. A feature of the underlying Large Language Model is that – much like a black box, there are always uncertainties – both statistical and systemic.
The term “hallucination” describes those times when AI presents incorrect information as fact. For example, I’ve been working with my colleagues at Fujitsu Research Europe on genomics AI. We built a model that can analyze genetic material such as skin samples for any signs of diseases; we compare subject samples with known good samples and determine whether the differences require further attention. More importantly, we provide the underlying reasoning. Out of curiosity we gave this same task to a Gen AI system, and it came back with a plausible explanation, but the outcome was factually incorrect. I use this for illustrative purposes: in the short to medium term at least, organizations need to use their own professional reviews and experience to validate the results.
Gen AI’s capabilities enable us to question the status quo and swiftly make groundbreaking discoveries, but we still need human knowledge and input to work alongside it. Put them together, and we can quickly test hypotheses that we may not have considered without the technology. So, see it as a way to augment your decision-making capability.
“People should be skeptical about trusting Gen AI. That's perfectly healthy. Given that there are quite a few factors to be concerned about, in the short to medium term businesses must have the right governance tools and human expertise reviewing and governing Gen AI.”
2. Boost trust and returns with clear use cases
“If AI is used appropriately, we will create a better world – I truly believe that. But people justifiably have their concerns about the technology, and are skeptical about trusting Gen AI systems.”
To incorporate Gen AI into their enterprises, business leaders should consider a multi-step process. From initial sandbox experimentation to the identification of appropriate use cases to custom intelligence discovery, the entire journey should be underpinned with expert review of the subject matter and appropriate security guardrails – for instance around personal data or key corporate data.
One way to help prevent Gen AI skepticism from reaching unhealthy levels in your business is to focus on clear use cases. In my experience, companies tend to have plenty of enthusiasm about AI, but are not clear about what they will use it for.
So ask yourself: what’s the specific business problem you need AI to solve? For instance, you might want to upskill your workforce or create lighter materials for your products. Choose a use case that’s likely to generate valuable returns for your company. Next, make sure you have access to the domain expertise – internally or externally – to verify the accuracy of your Gen AI outcomes. Start small and lean on human experience to monitor the systems and hopefully you will discover something new. This domain oversight will reduce skepticism and enable course correction of Gen AI tools to improve their performance over time.
3. Guard against algorithmic bias
Bias in input training data or algorithms can dramatically skew the integrity of Gen AI outputs. But detecting bias in training data is complex. At Fujitsu, we have an entire research unit in Europe and Israel working on detecting bias in AI training data.
It's impossible to completely eliminate risks such as bias, but it’s important to actively manage hazards in business. And there are several ways to tackle bias.
First, pull together a diverse multidisciplinary team to help inform and train your Gen AI systems. This will ensure that multiple perspectives are guiding how the tech is implemented and governed. These teams can, for instance, contribute to refining the guardrails embedded into Gen AI systems that teach the tool to avoid ethical issues such as unfair bias in its outputs.
Also consider recruiting an AI ethics specialist and using your technology specialists to routinely “red team” your Gen AI systems. Red teaming exercises stress-test the accuracy and moral code of your tools and you can use the learnings to upgrade your system guardrails.
And look to the wider industry for support. Some providers are experimenting with creating bias-management tools and embedding ethical codes and moral constitutions for Gen AI to operate with.
“Bias-management tools are going to become more important as Gen AI is implemented more widely.”
Stay focused on why and how Gen AI can benefit your business
To benefit from this transformative technology and use it responsibly, business leaders must focus on unraveling the range of risks and governance challenges associated with Gen AI. This means they need to:
-
See Gen AI as an assistant.
Gen AI requires expertise, relevant experience and lifecycle management to stay on the right track. Be alert to its limitations and work with them to gain business benefits.
-
Focus on the use case.
Instead of creating an AI that’s going to do everything for you, focus on what you really need. A contact center, for example, may focus on using Gen AI’s capability to sense users’ intent to expedite customer onboarding processes or address customer service enquiries.
-
Consider sustainability.
Some organizations are spending millions on building their own large language models to tap into Gen AI, which uses vast amounts of energy. Ask yourself whether it’s worth developing a new one yourself, or whether you could take advantage of existing technology such as LangChain and Fujitsu’s AI platform Kozuchi, and tune it to your needs.
For many organizations, rapid advances in Gen AI have the potential to transform almost every facet of life. To seize its opportunities, organizations will need to use Gen AI alongside their existing professional expertise – together with a healthy dose of skepticism.
John Walsh
VP of Technology, CTO Fujitsu Europe
John has over 30 Years’ experience in the technology industry, particularly Architecture, Consulting and Software development. He has held multiple roles within Fujitsu creating solutions with customers in Financial Services, Manufacturing, Law Enforcement, Retail, Telecommunications, and the Public Sector.
John has a keen interest in R & D having spent 3 years working on behalf of Fujitsu Laboratories in applied research (AI - ML, Graph Generation & Deep Tensor). This was followed by 2 years in Quantum inspired technologies and QUBO design. His current interest is in the combination of accelerating AI learning models using Quantum Techniques. He has a deep interest in the mathematics which underpins AI Ethics and the detection of bias in training data sets. He is an expert in the field of real-time computing and signalling processing.
John is at his best when given a business challenge and can apply technology to solve it, and a key element of his remit is to provide customers with early insight and adoption to Fujitsu Laboratory applied research.

Fujitsu Uvance
Innovative solutions that address business challenges and solve societal issues
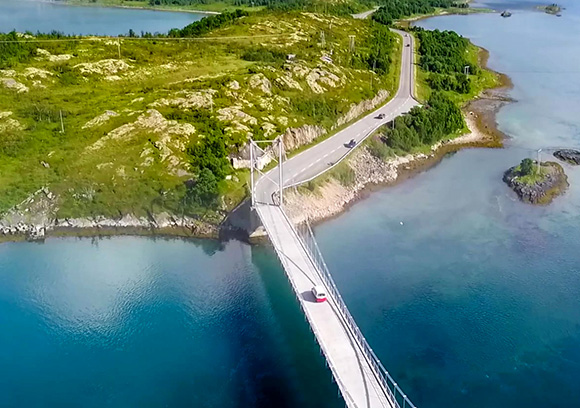
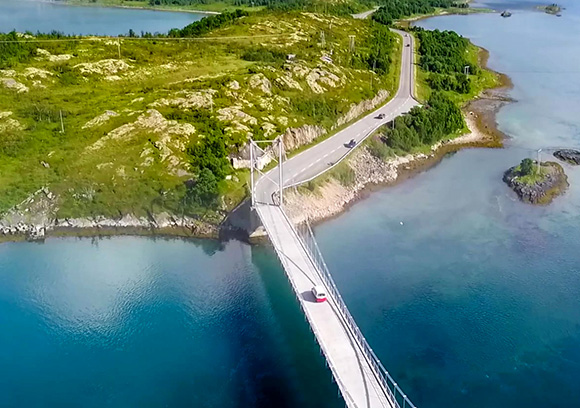
You may like
Business strategies for a connected world : Prospering in the digital society
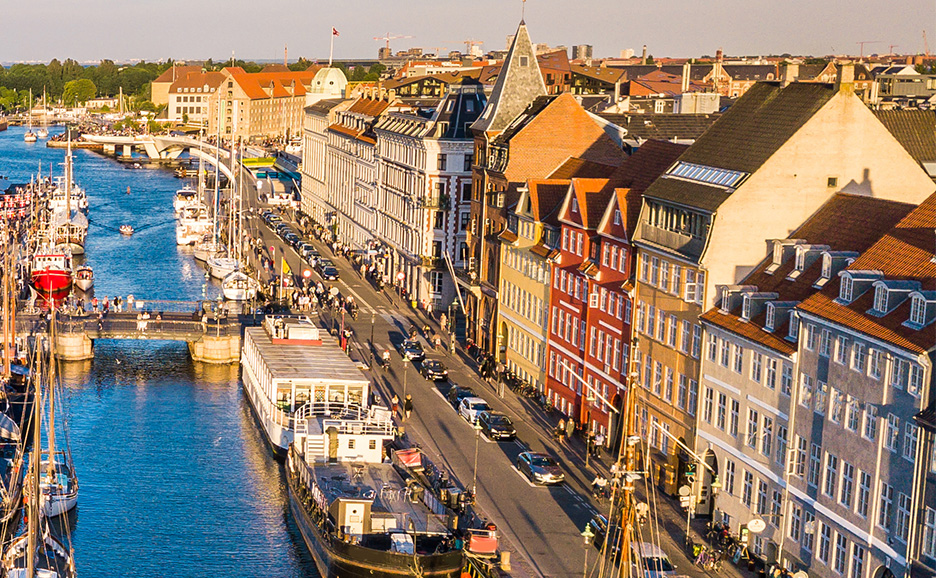
Our three guiding principles to unlocking sustainability success

A financially sustainable approach to carbon neutrality
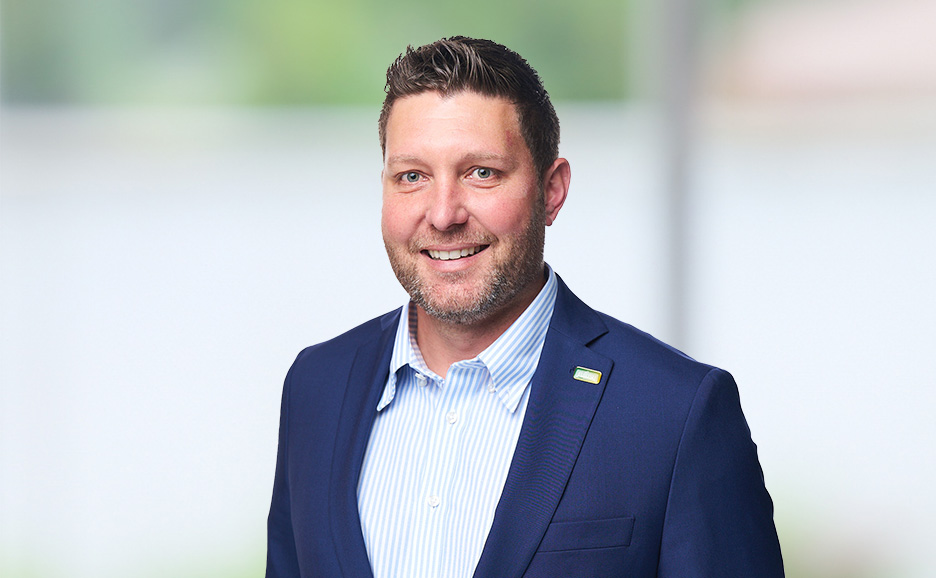
Happier employees can have a positive impact on your value chain
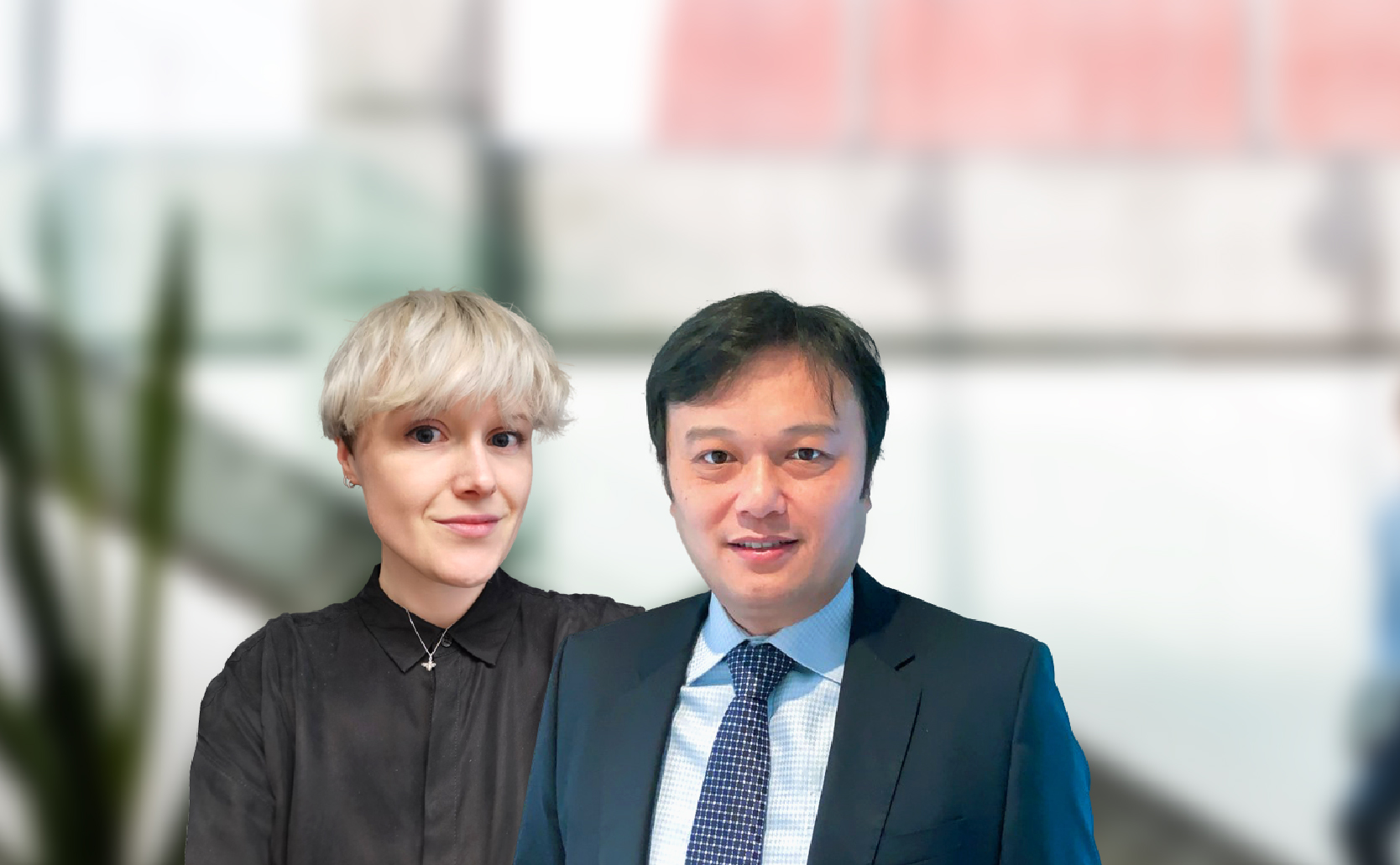
Pacific National:Data science identifying fleet wheel wear characteristics
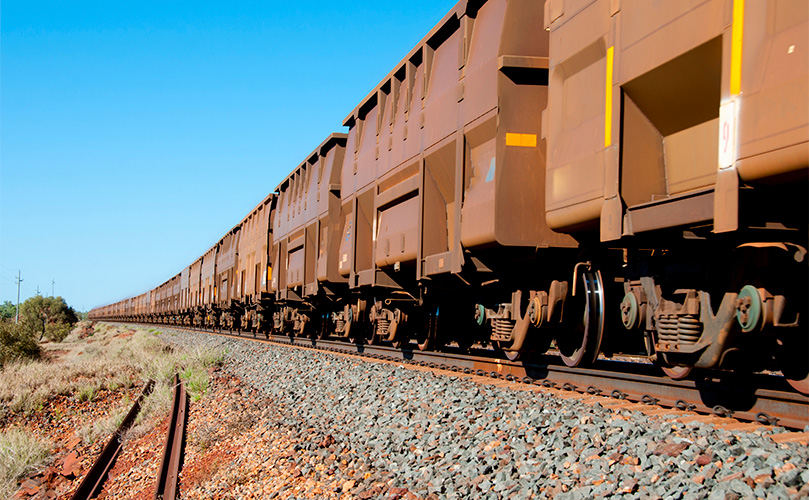